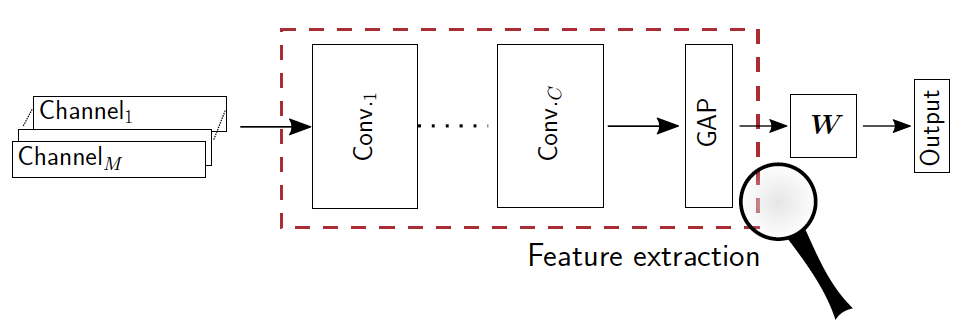
Validating Machine Learning-based Highly Automated Driving Functions by Diversity
The main focus of my doctoral project was researching ML safety methods used to build validation safety arguments of ML-based safety-critical driving functions. I built safety arguments by validating various aspects of deep neural networks. In my research, I focused on the following methods: representation learning, self- and semi-supervised learning, clustering methods, interpretability methods, and the distributional shifts between public highway driving datasets. I primarily worked with time-series data on object lists.